Looking at the development of the chip industry from four major trends.
This is the "Future Trends" series from MIT Technology Review, spanning various industries, trends, and technologies, taking you on a preview of the future.
Where is the future of chip manufacturing? With the convergence of tech giants, startup forces, AI technology, and global trade disputes, this question becomes particularly important. Tech giants are personally involved in chip making, while startups are seeking breakthroughs with innovative technologies, striving to gain an advantage in the chip battle of the intelligent era. The rise of edge computing is pushing AI closer to life, setting higher requirements for the miniaturization and high efficiency of chips. The competition for the chip supply chain between countries is reshaping the production and manufacturing landscape, striving for technological autonomy and security. The chip field is entering a new era of multi-party game-playing and continuous innovation.
Thanks to the vigorous development of the field of artificial intelligence, the chip industry is at a turning point of a huge wave. The market demand for chips that can train AI models more quickly and enable them to respond quickly between devices such as smartphones and satellites has surged, allowing users to use these models without leaking personal data. Governments, tech giants, and startups are all competing for the growing semiconductor market.
The following are several major trends that will define the next generation of chip shapes, producers, and new technologies within the next year.Countries Successively Introduce the "CHIPS Act"
Advertisement
On the outskirts of Phoenix, the world's two largest chip manufacturers, TSMC and Intel, are competing to build campuses in the desert, hoping to make it the new center of American chip manufacturing strength. The common point of these two efforts lies in the source of funding: In March 2022, U.S. President Biden announced the provision of $8.5 billion in direct federal funding and $11 billion in loans for Intel's expansion projects across the United States. A few weeks later, it was announced that TSMC would be provided with $6.6 billion in funding. These rewards are only a part of the U.S. subsidies that have been pouring into the chip industry through the 2022-signed $280 billion "CHIPS and Science Act." This funding means that any company involved in the semiconductor ecosystem is analyzing how to reorganize the supply chain to benefit from it. Although most of the funding is aimed at improving the U.S. chip manufacturing industry, other participants, including equipment manufacturers and specialized material startups, also have room to apply.
However, the United States is not the only country trying to localize part of the chip manufacturing supply chain. Japan is investing $13 billion in its own version of the "CHIPS Act," Europe plans to invest more than $47 billion, and India announced a $15 billion domestic chip factory construction plan earlier this year. The root of this trend can be traced back to 2014, according to Chris Miller, a professor at Tufts University and the author of "Chip War: The Battle for the World's Most Critical Technology," when China began to support domestic chip manufacturers.
"National governments of various countries are worried that they have no choice but to provide incentives, otherwise companies will transfer manufacturing to China," he said. This threat, coupled with the rise of AI, has prompted Western governments to fund alternatives. In the coming year, this may have a snowball effect, with more countries starting their own projects due to concerns about falling behind.
Miller pointed out that these funds are unlikely to lead to the emergence of new chip competitors or fundamentally reshape the landscape of the largest chip players. Instead, it mainly encourages leading companies like TSMC to establish a foothold in multiple countries around the world. However, funding alone is not enough to achieve this goal quickly—TSMC's efforts to build a factory in Arizona have been hindered by missed deadlines and labor disputes, and Intel has also failed to complete the construction plan as promised. Moreover, even if these factories eventually come online, it is uncertain whether their equipment and workforce will be able to match the advanced chip manufacturing capabilities of overseas counterparts."The reshaping and shifting of supply chains will be a slow process, taking years, even decades," Miller said, "but it is indeed undergoing a transformation."
New Advances in AI within Edge Computing
Currently, most of our interactions with AI models like ChatGPT are conducted through the cloud. This means that when you ask GPT to pick out clothing (or act as your boyfriend), your request first reaches OpenAI's servers, prompting the model residing there to process the request and come to a conclusion (known as "inference"), before sending the response back to you. Relying on the cloud has some drawbacks: it requires an internet connection and means that some of your data will be shared with the model's manufacturer.
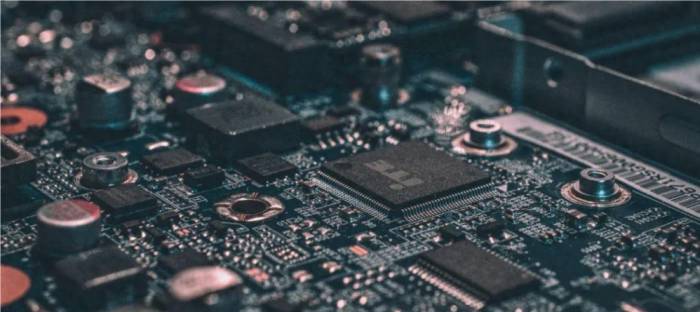
This is why there is a growing interest and investment in edge computing for artificial intelligence, where the process of invoking AI models is conducted directly on your device, such as a laptop or smartphone. As the industry continues to move towards AI models that understand us deeply (Sam Altman described to me his killer AI application that "completely understands my life, every email, every conversation I've ever had"), there is an increasing demand for faster "edge" chips that can run models without sharing private data. These chips face different constraints than those in data centers: they typically need to be smaller, cheaper, and more energy-efficient.
The U.S. Department of Defense is funding many studies on fast, private edge computing. In March 2022, its research arm, the Defense Advanced Research Projects Agency (DARPA), announced a collaboration with chip manufacturer EnCharge AI to create extremely powerful edge computing chips for AI inference. EnCharge AI is committed to developing a chip that can enhance privacy and operate with low power consumption. This will make it suitable for military applications, such as satellites and off-grid surveillance devices. The company is expected to ship these chips in 2025.AI models will continue to rely on the cloud in some application scenarios, but new investments and interest in edge computing improvements may bring faster chips to our everyday devices, enabling more AI applications. If edge chips become small and cheap enough, we are likely to see more AI-driven "smart devices" at home and in the workplace. Today, AI models are mostly confined within data centers.
Naveen Verma, co-founder of EnCharge AI, said, "Many of the challenges we see in data centers will be overcome. I anticipate that edge computing will become a focus, and I believe this is crucial for the large-scale deployment of AI."
Large tech companies enter the chip manufacturing competition.
Companies in various industries, from fast fashion to lawn care, are paying high computing costs to create and train AI models suitable for their businesses. For example, models that employees can use to scan and summarize documents, as well as external technologies such as virtual assistants that can help you repair a damaged refrigerator. This means that the demand for cloud computing required to train these models is unprecedented.The companies that provide most of this computational power are Amazon, Microsoft, and Google. For years, these tech giants have been dreaming of increasing their profit margins by manufacturing their own data center chips instead of purchasing them from companies like Nvidia. Nvidia has almost monopolized the market for the most advanced AI training chips, with a market value exceeding the GDP of more than 183 countries.
Amazon began trying this in 2015, with the key move being the acquisition of the startup Annapurna Labs. Google followed in 2018 with the launch of its own TPU chips. Microsoft launched its first AI chip in November last year, and Meta showcased its latest version of AI training chips in April this year.
This trend may weaken Nvidia's position. However, in the eyes of large tech companies, Nvidia is not only a competitor: no matter how much they internally strive, cloud giants still need Nvidia's chips to meet the needs of their data centers. This is partly because their own chip manufacturing efforts cannot meet all the needs, and also because their customers expect to be able to use top-notch Nvidia chips.
Rani Borkar, head of Azure hardware at Microsoft, said, "This is actually about giving customers the right to choose." She said she could not imagine a future where Microsoft would provide all the chips for its cloud services: "We will continue to strengthen the partnership and deploy chips from all chip partners."
As cloud computing giants try to compete for market share from chip manufacturers, Nvidia is also trying to go against the trend. Last year, the company launched its own cloud service, allowing customers to bypass Amazon, Google, or Microsoft and directly get computing time on Nvidia chips. As this fierce competition for market share unfolds, the next year will be a key period to test whether customers will consider the chips of large tech companies as equivalent to Nvidia's most advanced chips, or more like their smaller versions.NVIDIA and the Startups' Competition
Despite NVIDIA's dominant position, a wave of investment is pouring into those startups aiming to surpass NVIDIA in some specific segments of the chip market's future. These startups all promise faster artificial intelligence training speeds, but they each have their own claims about which dazzling computing technology to use to achieve this goal, ranging from quantum computing to photonics to reversible computing.
However, Murat Onen, the 28-year-old founder of the chip startup Eva, who has transformed this company from his doctoral research at the Massachusetts Institute of Technology, is outspoken about his feelings on starting a chip company at the moment.
"In this field, NVIDIA is the king of the mountain, and that's the reality we are in," he said.
Many companies like SambaNova, Cerebras, and Graphcore are trying to change the basic architecture of the chip. Imagine an AI acceleration chip that needs to constantly transfer data between different areas: information is stored in the memory area, then it must be moved to the processing area for computation, and then stored back in the memory area to save. All of this consumes time and energy.Optimizing this process will bring faster and more cost-effective AI training to customers, but this can only be achieved if chip manufacturers have excellent software that allows AI training companies to transition seamlessly to new chips. If the software migration process is too clumsy, model vendors like OpenAI, Anthropic, and Mistral are likely to continue relying on large chip manufacturers. This means that companies adopting this strategy, such as SambaNova, will have to spend a lot of time on chip design and also invest a lot of effort in software design.
Onen proposes a more profound change. Instead of using traditional transistors (which have achieved higher efficiency through decades of miniaturization), he has adopted a new component called the proton-gated transistor, which is said to be specifically designed by Eva to meet the mathematical needs of AI training. This allows data to be stored and processed in the same location, saving time and computational energy. The idea of using such components for AI inference can be traced back to the 1960s, but due to material barriers and other reasons, researchers have always failed to solve the problem of how to apply them to AI training, one of the key requirements being the precise control of conductivity at room temperature.
One day in the lab, "by optimizing these parameters, coupled with good luck, we got the material we wanted," said Onen. "Suddenly, this device is no longer like a science fair project." This has increased the possibility of large-scale use of such components. After months of work to confirm the data was correct, he founded Eva, and the related work was published in Science.
But in this industry, many founders have promised - but failed - to overthrow the dominance of leading chip manufacturers. Onen frankly admits that it will take years to determine whether the design works as expected and whether manufacturers will agree to produce it. He said that leading the company forward in such uncertainty requires flexibility and a willingness to accept others' questioning.
"Sometimes, I think people are too attached to their own ideas, worried that once this idea fails, there will be no next step," he said, "I don't think so. I still hope someone will challenge us and tell us this is wrong."